Implementing your research project: analysing your data
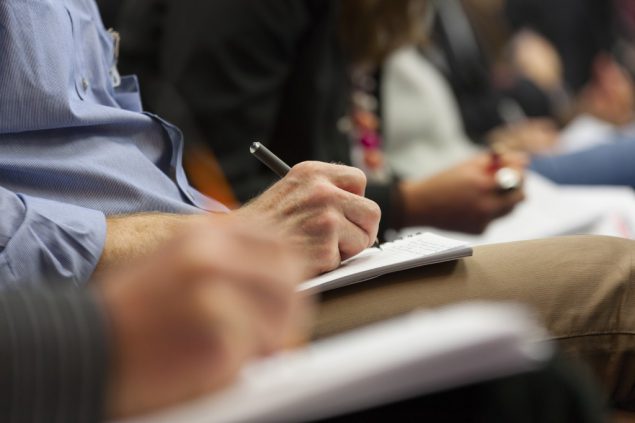
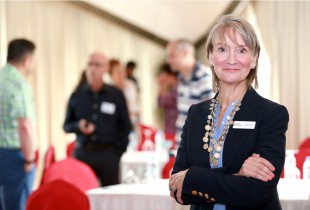
Once you have collected your information you will need to analyse it. In this blog I will be looking at two broad methods of data analysis – quantitative (numbers) and qualitative (words). Then I will comment on drawing conclusions from your data.
1. Quantitative data analysis.
First, to say that sophisticated statistical analysis should probably best be left to sophisticated statisticians! If your research project includes large data sets based on random samples looking for proof of generalisable ‘cause and effect’ relationships or statistical modelling this is probably best done with the advice of a specialist in this area – who will already have advised you on your research question, research design and methodology.
Quantitative analysis is basically counting – how many, how much, how often, how long – and aims to be logical, and objective – although this depends on how objectively your data are collected, coded and allocated. In this section I will be providing a brief overview of some types of numerical data and look at simpler analysis which would be relevant for organisational or survey data such as sickness absence rates, staff turnover, customer complaints, sales figures, educational achievement of students or health outcomes for patients. The focus will be on ‘descriptive statistics’ and graphical presentation of data.
Numerical data can either be ‘categorical’ or ‘quantifiable’.
Categorical data can be classified into groups and a number allocated – for example, gender. In this case, you could allocate ‘1’ to subjects who are Male, and ‘2’ to subjects who are Female. Once these values have been allocated to each subject correctly, data can be inputted into a simple spreadsheet such as Excel and the proportion of male to female identified. Analysis can then describe, say, the proportion of male to female participants who take up the offer of coaching training in an organisation or the proportion of male to females who are being or have been coached.
Quantifiable numbers are real numerical values which can be ranked in order – continuous data can take any point in a range, for example length of service in an organisation; discrete data can be measured precisely, for example number of clients coached or number of customers served.
The first step towards completing your data analysis is coding. This may be done as part of the data collection, as when a survey asks you to select an answer which most applies to you, e.g. what age are you? Are, more usually, which of a predetermined age group do you belong to? Coding simply refers to allocating a single number to a variable or factor – this might be the actual number, e.g. actual income of a subject; or a number allocated to a grouping of income. For the former, an example of income might be £21,500; and of an income group, £20,000 – £29, 999 – to which the actual income would be allocated in this example. It is helpful to use established, published coding frames where available and relevant – e.g. classification of ethnic status, social class or marital status as coded by the Office of Population Censuses and Survey.
Once coded the next step is to input your data into the computer and software programme of your choice. Once entered, it is crucial to check for errors – in either the coding or the data entry. This can be very time-consuming, but deciding not to undertake it can be dangerous, as errors (there are always errors!) will feed through into the analysis and findings, and from there lead you to false conclusions.
The first stage of analysis is usually about exploring the data you have collected and looking for findings of interest. For evaluating the effectiveness of coaching in an organisation you may wish to look at the distribution of different age groups compared to the benefit they perceive they have received from coaching, or the number of coaching sessions research subjects have received compared to the number of days lost to sickness/absence. The easiest way to start understanding the data is through the use of tables and, particularly, diagrams such as bar charts (to show the frequency of occurrences from highest to lowest), pie charts (to show the proportion of distribution of variables) or scattergrams (to show the relationship between two variables). Graphic presentation of data can now easily and speedily be generated by everyday software. Descriptive statistics enable numerical descriptions and comparisons of variables, and focus on the spread of the variable across your subjects; and the tendency of the data to group towards the centre. Examples of the latter include the mode (the value that occurs most frequently), the mean or average, and the median (which represents the middle value). Measures of the spread of data include for example: the range (difference between the highest and lowest); the inter-quartile range (the difference within 50% of values) and the standard deviation (the extent to which data differ from the average).
A word of warning about the ease in which numerical data can be analysed by spreadsheets such as ‘Excel’. This software (and others like it) can readily be used to present tables, graphs of various sorts and quite complex statistical analyses. Be aware that, although this software can produce sophisticated graphs and statistics these are only as valid and useful as the quality of data input and the correctness of the function requested of the programme – or, the risk is, as the acronym GIGO has it, Garbage In, Garbage Out, even if it is beautifully presented!
Reference: M Saunders, P Lewis, A Thornhill (2000) Research Methods for Business Studies 2nd edition, Chapter 11. This includes more technical detail and more information about statistical analysis.
2. Qualitative data analysis
Many of us are more comfortable with words than numbers so there is a tendency to see qualitative data analysis as easy – which is not necessarily the case. You still need to take care that you can ensure that your conclusions are based on the evidence you have collected.
A common method of qualitative data analysis is ‘categorisation’ – or coding – which breaks down the data into meaningful and related parts (categories) allowing you to identify key themes from different sources (e.g. interview transcripts or policy documents). These categories enable you to manage your data and bring together concepts and ideas from the data. Coding can be done manually (post-it notes, cutting and pasting from interview transcripts) or electronically (creating a coding table or using computer software designed for qualitative analysis). Coding is an iterative process and is usually done as you go along, after each interview for example, checking all the while that your themes or concepts accurately reflect the data you have collected. Re-analysing earlier interview data in the light of categories or themes emerging from later interviews can enhance and deepen your understanding of the data and its significance; or you may wish to re-catergorise earlier interviews in the light of understanding developed in the later interviews. It can also be helpful for you to keep a record of ideas and any other information such as the context, individuals involved or non-verbal cues as you go along. Keeping summaries of key points from each interview can also help you to identify emerging themes and categories.
It is probably more usual to use an inductive approach to analysing qualitative data – that is, you seek to build up theory and understanding derived from and grounded in the data you collect (rather than a deductive approach which involves starting with a theory or hypothesis then collecting data to test the theory’s validity). This inductive approach allows your research participants to ‘drive’ the development of theory and avoids the risk that their views would be forced into a theory or interpretation which they themselves would disavow. It can be useful to quantify qualitative data – i.e to count the number of times a particular event, issue or theme occurs in the course of your data collection and present this as a table.
3. Drawing conclusions from your data analysis.
Whichever type of data you collect and analyse, great care must be taken to ensure that the conclusions you draw from the data are sound and accurate. Review your data and analysis carefully and check that you have relevant and appropriate evidence for your conclusions to the research. For example, a small survey of staff who have been on a coaching programme is unlikely to ‘prove (or disprove) that coaching is effective’. It may however indicate, that for those staff involved, they perceived that their engagement and motivation at work, and hence their performance, were improved. This could, however, inform a larger study, with measurable outcomes to formally evaluate the effectiveness of coaching in the workplace. Alternatively, a large questionnaire survey could provide evidence of effectiveness of coaching but would be less likely to explore the staff’s perceptions and experiences of coaching and being coached.
Reference: M Saunders, P Lewis, A Thornhill (2000) Research Methods for Business Studies 2nd edition, Chapter 12. This also includes more technical detail and information.